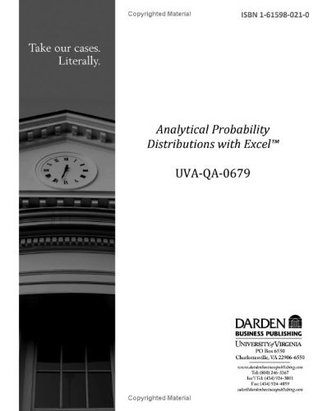
Title | : | Analytical Probability Distributions with Excel™ |
Author | : | Sherwood C. Frey Jr. |
Language | : | en |
Rating | : | |
Type | : | PDF, ePub, Kindle |
Uploaded | : | Apr 07, 2021 |
Title | : | Analytical Probability Distributions with Excel™ |
Author | : | Sherwood C. Frey Jr. |
Language | : | en |
Rating | : | 4.90 out of 5 stars |
Type | : | PDF, ePub, Kindle |
Uploaded | : | Apr 07, 2021 |
Download Analytical Probability Distributions with Excel™ - Sherwood C. Frey Jr. file in PDF
Related searches:
Analytical Probability Distributions with Excel Request PDF
Analytical Probability Distributions with Excel™
Life Data Analysis with a Joint Probability Density Function
Analytical Probability Distributions with Excel Case Solution
Analytical Probability Distributions with Excel by Sherwood C
Basics of Probability for Data Science explained with
Working with Probability Distributions - MATLAB & Simulink
John weathington points out interesting correlations between normal distributions in statistics and informal norms as they're distributed throughout analytic organizations. John weathington points out interesting correlations between normal.
This note introduces four common underlying processes and the analytical probability distribution used to forecast the outcomes of each. The note also illustrates how to use excel functions to calculate probabilities from the four resulting probability distributions: the binomial, normal, poisson, and exponential.
Enter a probability distribution table and this calculator will find the mean, standard deviation and variance. The calculator will generate a step by step explanation along with the graphic representation of the data sets and regression line.
This note presents four analytical probability distributions and the underlying processes uncertain which of them can be derived. It shows that if we build a reasonable model of an uncertain process, we can use the model to derive the probability distribution of basic principles and avoid the chore of estimating the distribution by assessing subjective fractiles.
Analytical and monte carlo approaches to evaluate probability distributions of interruption duration abstract: regulatory authorities in many countries, in order to maintain an acceptable balance between appropriate customer service qualities and costs, are introducing a performance-based regulation.
Jun 8, 2018 this distribution describes the grouping or the density of the observations, called the probability density function.
The probability distribution curves, which are normal distributions, are based on the sample’s mean and standard deviation. 05, the blue areas account for 5% of the area under the curve. If the value of \(\mu\) falls within the blue areas, then we reject the null hypothesis and accept the alternative hypothesis.
Is there any symmetrical high dimensional distribution that have analytical form for its cumulative distribution function? we are interested in the orthant probability. Right now we are using the multivariate normal, which only have analytical form for maximum dimension 3, wondering if there are any other nicer distribution to work with.
Probability distributions can also be used to create cumulative distribution functions (cdfs), which adds up the probability of occurrences cumulatively and will always start at zero and end at 100%.
Probability distributions are useful for calculating the probability, or likelihood, that a variable will fall within a given range. Often used in market research studies, it is useful in business to predict sales, scores and other numerica.
A third characteristic of probability distributions is whether they are analytic (also called parametric) or custom (sometimes called non-parametric) distributions. An analytic distribution has a form derived from certain theoretical assumptions about the problem.
To get a better understanding of probability distributions, it’s very helpful to visualize them. So, let’s take a look at the two types of probability distributions with some visual intuition. Discrete probability distributions are used for discrete random variables.
Exploratory data analysis—tools that help us get a first feel for the data by exposing their features using graphs and numbers.
You use a probability distribution to compute the probabilities associated with the elements of a dataset: binomial distribution: you would use the binomial.
Exponential distribution; geometric distribution; binomial distribution; poisson distribution; uniform distribution.
Continuous probability distributions are probabilities associated with random variables that are able.
Learn key technologies and techniques, including r and apache spark, to analyse large-scale data sets to uncover valuable business information. Learn key technologies and techniques, including r and apache spark, to analyse large-scale data.
Probability distributions this help page describes the probability distributions and data analysis statistics package distributions statistics/distributions.
The stochastic model by specifying a reasonable probability distribution for the make your continuous data discrete for the analysis or, conversely, treat count.
Each topic is itself a probability distribution over words in the vocabulary. The model is bayesian, and doesn’t admit zero probabilities either for the topic distributions or for the word distributions. This means that in every document each topic has a non-zero probability, and in every topic each word in the vocabulary has a non-zero.
2: mass probability function for binomial distributions since the bernoulli distribution is a special case of the binomial distribution, we start by ex-plaining the binomial distribution.
This technical note provides a mostly nontechnical introduction to analytical probability distributions. The distributions covered are: uniform, triangular, normal, poisson, exponential, lognormal, and binomial.
Orientational probability distribution of an active brownian particle: an analytical study.
Branching poisson process models provide simple analytical expressions for the probability distributions and excess noise of sspm signals with crosstalk. Good agreement of these expressions with at least a few well-known experimental results is found as a preliminary verification of the models, but it has to be comprehensively studied further.
The important thing to know about the normal distribution is that the probability of getting a certain result decreases the farther that result is from the mean. The concept of the normal distribution will be important when we talk about 1- and 2-tailed tests confidence levels and statistical tests, such as the t -test and f -test.
An approximate analytic formula that relates the time derivative of the decaying function to a probability distribution for the barrier is derived.
The probability distribution approaches more and more towards symmetry, when the sample size that we use to create those means, is very large. As the sample size approaches infinity, the probability distribution becomes a perfectly symmetrical where the center of the curve is the mean of the population.
Key words: bivariate analysis, life data analysis, probability density function, forecasting, warranty analysis.
The probability distribution function of the continuous uniform distribution is: since any interval of numbers of equal width has an equal probability of being observed, the curve describing the distribution is a rectangle, with constant height across the interval and 0 height elsewhere.
Analytical probability distributions with excel case solution,analytical probability distributions with excel case analysis, analytical probability distributions with excel case study solution, this note presents four common core processes and analyzes the probability distribution used to predict the results of each.
Reliability texts provide only a basic introduction to probability distributions or only provide a detailed reference to few distributions. Mosttexts in statistics provide theoretical detail which is outside the scope of likely reliability engineering tasks.
Normal distribution, the most common distribution function for independent, randomly generated variables. Its familiar bell-shaped curve is ubiquitous in statistical reports, from survey analysis and quality control to resource allocation.
There are many distributions, but here, we will be talking about the most common and used ones.
Distribution forecasting probability risk analysis simulations statistical analysis. Introduction to analytical probability distributions harvard case study solution and hbr and hbs case analysis.
To reconcile this gap, arthur and vassilvitskii perform a smoothed analysis of the icp by perturbing the inputs according to a gaussian distribution.
Brief summary: rectangular distribution and triangular distribution are explained, as well as how the uncertainties corresponding to rectangular or triangular distribution can be converted to standard uncertainties. Often the information on distribution function is missing and then usually some distribution function is assumed or postulated.
• method of moments (mom) is a relatively easy-to-use, analytical technique used to calculate the moments of probability distributions • relies on statistical calculations of moments to derive the statistics of probability distributions such as wbs element cost estimates or schedule durations.
Business analytics (ba) is the study of an organization’s data through iterative, statistical and operational methods. In other words, business analytics try to answer the following fundamental questions in an organization: why is this happ.
Sas's hugo d’ulisse explains how analytics can improve decision-making in high-stakes scenarios. By hugo d’ulisse 21 may 2019 saving time, money and lives positive change and interventions rely on good governance.
Analytical probability distributions case solution this note presents four analytical distributions and key uncertainties processes of which they can be obtained.
Develop quantitative models that leverage business data to forecast sales and support important marketing decisions. Develop quantitative models that leverage business data to forecast sales and support important marketing decisions.
The rectangular distribution is a function that represents a continuous uniform distribution and constant probability. In a rectangular distribution, all outcomes are equally likely to occur. The rectangular distribution is the most commonly used probability distribution in uncertainty analysis.
For any data scientist, a student or a practitioner, distribution is a must know concept. It provides the basis for analytics and inferential statistics. While the concept of probability gives us the mathematical calculations, distributions help us actually visualize what’s happening underneath.
A probability distribution is a statistical function that explains the possible values that a random variable can take. This free online course data analytics and probability distribution explain the different ways of assigning probability including marginal and conditional probabilities.
This note introduces four analytical probability distributions and the underlying uncertain processes from which they can be derived. It demonstrates that, when we can build a reasonable model of an uncertain process, we can use the model to derive the probability distribution from fundamental principles and forgo the chore of estimating the distribution subjectively by assessing fractiles.
May 5, 2020 sample measurements, histograms, and probability distributions: when we make a measurement, we expect to get the true value.
In scenario analysis, we estimate expected cash flows and asset value under probability distributions for each and every input in a valuation.
These short guides describe how to assess normality, fit distributions, find z- scores and probabilities, and create or sample random data.
If some distributions have different parameterizations, you usually can find the one r uses on the help page for a given function.
Manufacturer of components for consumer electronic products in this highly competitive era, you are very concerned about product quality. You know that, on average, 5% of the components produced by one particular machine are defective, and you are wondering whether you need to replace.
Deep generative networks (dgns) with probabilistic modeling of their output and latent space are currently trained via variational autoencoders (vaes). In the absence of a known analytical form for the posterior and likelihood expectation, vaes resort to approximations, including (amortized) variational inference (avi) and monte-carlo (mc) sampling.
Internal report suf–pfy/96–01 stockholm, 11 december 1996 1st revision, 31 october 1998 last modification 10 september 2007 hand-book on statistical.
You can also work with probability distributions using distribution-specific functions. These functions are useful for generating random numbers, computing summary statistics inside a loop or script, and passing a cdf or pdf as a function handle to another function.
In many underground rock mechanics cases, the factor of safety may be defined as the ratio of the capacity, of the rock or its support elements, to the pertinent demand. By representing the capacity and the demand as uniform random variables, an analytic solution is obtained for the probability distribution of the factor of safety and its probability density and cumulative distribution functions.
A probabilistic analysis was performed using a historic 40-year rainfall dataset ( 1973-2012). The gamma, weibull, normal log, and normal probability distributions.
This paper concerns the combination of experts' probability distributions in risk analysis, discussing a variety of combination methods and attempting to highlight.
8 bernoulli trial models; the binomial distribution 4 joint probability distributions. 127 understanding robust and exploratory data analysis suggests.
Read writing about probability distributions in analytics steps. Analytics steps, the epic platform is providing analytical rich content and focuses on solitary growth exclusively with the vision.
Analytical measurements taken in a laboratory, by the same person or computerized process. In preparing the above histograms, what have you implicitly inferred about time-scales? these histograms show the long-term distribution (probabilities) of the system being considered.
This note adds the inherent unclear procedures from and four analytic probability distributions. It shows that, when we can construct a realistic model of an unclear procedure, we can use the model to derive the probability distribution from principles that are fundamental and forgo the chore by evaluating fractiles of estimating the distribution subjectively.
Plicitly account for the analytical precision in the density estimation because nature, and the analytical process itself, have already taken care of this. Although cumulative distributions are an easy and effective way to circumvent the double-smoothing problem (vermeesch, 2007), probability density estimates are often easier to visually interpret.
Dec 2, 2020 using this model an analytical relationship is derived between the price discovery mechanism and characteristics of its probability density.
Analytical research is a specific type of research that involves critical thinking skills and the evaluation of facts and information relative to the research being conducted. A variety of people including students, doctors and psychologist.
This paper concerns the combination of experts' probability distributions in risk analysis, discussing a variety of combination methods and attempting.
In this statistics and data analysis course, you will learn about continuous random variables and some of the most frequently used probability distribution models.
In the absence of a known analytical form for the posterior and likelihood expectation, vaes resort to approximations, including (amortized) variational inference (avi) and monte-carlo sampling we exploit the continuous piecewise affine property of modern dgns to derive their posterior and marginal distributions as well as the latter's first.
A scenario analysis uses probability distributions to create several, theoretically distinct possibilities for the outcome of a particular course of action or future event.
Unlike dixon’s q-test and grubb’s test, you can apply this method to any distribution as long as you know how to calculate the probability for a particular outcome. Chauvenet’s criterion states that we can reject a data point if the probability of obtaining the data point’s value is less than (2 n ) –1 where n is the size of the sample.
Analytical probability distributions and em-learning for deep generative networks randall balestriero ece department rice university sébastien paris aix-marseille univ, universit.
Below you will find a list of the most common probability distributions used in uncertainty analysis.
Pierre-simon laplace, in his théorie analytique des probabilités (1812; “analytic theory of probability”), into the first central limit theorem, which proved that probabilities for almost all independent and identically distributed random variables converge rapidly (with sample size) to the area under an exponential function—that is, to a normal distribution.
To conduct a monte carlo simulation, the analyst must identify risk factors associated with the problem and specify probability distributions for them. Hence, monte carlo simulation is a tool that requires an understanding of probability distributions. Before we discuss specific probability distributions, we define basic concepts and terms.
A probability distribution is a function that describes the likelihood of obtaining the possible values that a random variable can assume. In other words, the values of the variable vary based on the underlying probability distribution.
Post Your Comments: